Introdution to TAO and TAO Toolkit
Nvidia Train Adapt Optimize (TAO) Toolkit is a Python based AI toolkit for development of purpose-built AI models and customizing them with users' own data.
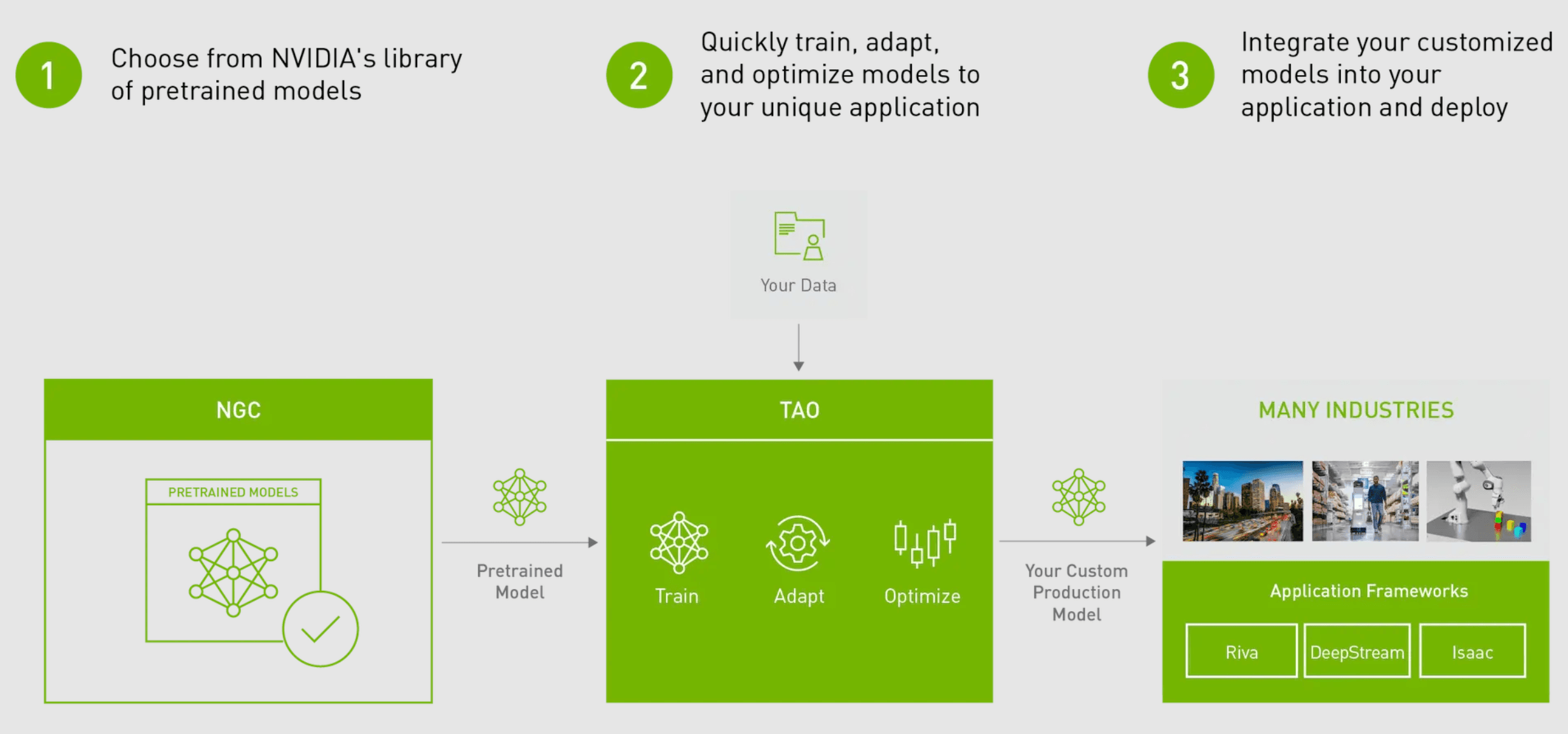
The overall architecture consists of two parts Nvidia-TAO (Frontend) and Nvidia Tao Toolkit (Backend).
TAO
TAO is a is a GUI based AI-model-adaptation framework that simplifies and accelerates the creation of enterprise AI applications and services. Official Site: Nvidia TAO
TAO Toolkit
TAO Toolkit is a CLI and Jupyter notebook based solution that simplifies the task of transfer learning and fine-tuning on computer vision and conversation AI models. The NVIDIA TAO Toolkit simplifies this process by abstracting away the AI/DL framework complexity. Official Site: Nvidia TAO Toolkit
Action Recognition with TAO Toolkit
We will use jupyter notebook for running the code shown in this tutorial.
Create a folder named workspace. We will use this as the root of our project
%env HOST_DATA_DIR=/home/user/AJC/workspace/action_recognition_net/data # Absolute path to directory to store the training data %env HOST_SPECS_DIR=/home/user/AJC/workspace/action_recognition_net/specs # Absolute path to directory to store the training specs %env HOST_RESULTS_DIR=/home/user/AJC/workspace/action_recognition_net/results # Absolute path to directory to store the training output %env KEY = nvidia_tao # Encryption key of nvidia model. No need to change this.
Let's create the sub directories
!mkdir -p $HOST_DATA_DIR !mkdir -p $HOST_SPECS_DIR !mkdir -p $HOST_RESULTS_DIR
Create a tao_mounts.json file which mounts the specified directories in TAO docker
import json import os mounts_file = os.path.expanduser("~/.tao_mounts.json") tlt_configs = { "Mounts":[ { "source": os.environ["HOST_DATA_DIR"], "destination": "/data" }, { "source": os.environ["HOST_SPECS_DIR"], "destination": "/specs" }, { "source": os.environ["HOST_RESULTS_DIR"], "destination": "/results" }, { "source": os.path.expanduser("~/.cache"), "destination": "/root/.cache" } ], "DockerOptions": { "shm_size": "16G", "ulimits": { "memlock": -1, "stack": 67108864 } } } # Writing the mounts file. with open(mounts_file, "w") as mfile: json.dump(tlt_configs, mfile, indent=4)
!cat ~/.tao_mounts.json
Installing Pre-Requisities
The basic requirements required for this tutorial is as following
- python >=3.6.9 < 3.8.x
- docker-ce > 19.03.5
- docker-API 1.40
- nvidia-container-toolkit > 1.3.0-1
- nvidia-container-runtime > 3.4.0-1
- nvidia-docker2 > 2.5.0-1
- nvidia-driver > 455+
Once you have installed the pre-requisites, please log in to the docker registry nvcr.io
docker login nvcr.io
You will be asked to enter a username and password. The username is $oauthtoken and the password is the API key generated from ngc.nvidia.com. Please follow the instructions in the NGC setup guide to generate your own API key.
Now install the other required libraries.
!pip3 install nvidia-pyindex # Nvidia PIP Index !pip3 install nvidia-tao # Nvidia TAO Toolkit library !apt update !apt-get install unrar # Required for extracting downloaded data !pip3 install xmltodict opencv-python # Required for dataprocessing
To verif the setup, run
!tao info
The result will be like this
Configuration of the TAO Toolkit Instance dockers: ['nvidia/tao/tao-toolkit-tf', 'nvidia/tao/tao-toolkit-pyt', 'nvidia/tao/tao-toolkit-lm'] format_version: 2.0 toolkit_version: 3.21.11 published_date: 11/08/2021
Preparing the Dataset
We are using HMDB51 Dataset to finetune the action recognition model.
!wget -P $HOST_DATA_DIR http://serre-lab.clps.brown.edu/wp-content/uploads/2013/10/hmdb51_org.rar # Downloads the dataset !mkdir -p $HOST_DATA_DIR/videos && unrar x $HOST_DATA_DIR/hmdb51_org.rar $HOST_DATA_DIR/videos #Unrar downloaded data !mkdir -p $HOST_DATA_DIR/raw_data #Directory to copy the data to
Extract the required classes into $HOST_DATA_DIR/raw_data folder.
!unrar x $HOST_DATA_DIR/videos/fall_floor.rar $HOST_DATA_DIR/raw_data !unrar x $HOST_DATA_DIR/videos/ride_bike.rar $HOST_DATA_DIR/raw_data
Download dataset preprocessing script
!git clone https://github.com/NVIDIA-AI-IOT/tao_toolkit_recipes
Run the preprocessing
!cd tao_toolkit_recipes/tao_action_recognition/data_generation/ && bash ./preprocess_HMDB_RGB.sh $HOST_DATA_DIR/raw_data $HOST_DATA_DIR/processed_data
Download the official train-test split from HMDB site
!cd $HOST_DATA_DIR && wget http://serre-lab.clps.brown.edu/wp-content/uploads/2013/10/test_train_splits.rar !cd $HOST_DATA_DIR && mkdir splits !unrar x $HOST_DATA_DIR/test_train_splits.rar $HOST_DATA_DIR/splits
Split the downloaded dataset based on the train-test split
!cd tao_toolkit_recipes/tao_action_recognition/data_generation/ && python3 ./split_dataset.py $HOST_DATA_DIR/processed_data $HOST_DATA_DIR/splits/testTrainMulti_7030_splits $HOST_DATA_DIR/train $HOST_DATA_DIR/test
Downloading Pre-Trained Model from Nvidia NGC
Installing NGC CLI on the local machine.
import os %env CLI=ngccli_cat_linux.zip !mkdir -p $HOST_RESULTS_DIR/ngccli # Remove any previously existing CLI installations !rm -rf $HOST_RESULTS_DIR/ngccli/* !wget "https://ngc.nvidia.com/downloads/$CLI" -P $HOST_RESULTS_DIR/ngccli !unzip -u "$HOST_RESULTS_DIR/ngccli/$CLI" -d $HOST_RESULTS_DIR/ngccli/ !rm $HOST_RESULTS_DIR/ngccli/*.zip os.environ["PATH"]="{}/ngccli:{}".format(os.getenv("HOST_RESULTS_DIR", ""), os.getenv("PATH", ""))
Find the Action Recognition Model on Nvidia NGC
!ngc registry model list nvidia/tao/actionrecognitionnet:*
The output will be like this
+-------+-------+-------+-------+-------+-------+-------+-------+-------+ | Versi | Accur | Epoch | Batch | GPU | Memor | File | Statu | Creat | | on | acy | s | Size | Model | y Foo | Size | s | ed | | | | | | | tprin | | | Date | | | | | | | t | | | | +-------+-------+-------+-------+-------+-------+-------+-------+-------+ | train | 88.0 | 120 | 1 | V100 | 426.2 | 426.1 | UPLOA | Nov | | able_ | | | | | | 6 MB | D_COM | 23, | | v1.0 | | | | | | | PLETE | 2021 | | deplo | 90.0 | 120 | 1 | V100 | 170.3 | 170.3 | UPLOA | Oct | | yable | | | | | | 3 MB | D_COM | 22, | | _v1.0 | | | | | | | PLETE | 2021 | +-------+-------+-------+-------+-------+-------+-------+-------+-------+
Download the Pre-trained Model
!mkdir -p $HOST_RESULTS_DIR/pretrained # Pull pretrained model from NGC !ngc registry model download-version "nvidia/tao/actionrecognitionnet:trainable_v1.0" --dest $HOST_RESULTS_DIR/pretrained
Add the configuration file to $HOST_SPECS_DIR with the filename train_rgb_3d_finetune.yaml
output_dir: /results/rgb_3d_ptm encryption_key: nvidia_tao model_config: model_type: rgb backbone: resnet18 rgb_seq_length: 3 input_type: 3d sample_strategy: consecutive dropout_ratio: 0.0 train_config: optim: lr: 0.001 momentum: 0.9 weight_decay: 0.0001 lr_scheduler: MultiStep lr_steps: [5, 15, 20] lr_decay: 0.1 epochs: 20 checkpoint_interval: 1 dataset_config: train_dataset_dir: /data/train val_dataset_dir: /data/test label_map: fall_floor: 0 ride_bike: 1 output_shape: - 224 - 224 batch_size: 32 workers: 8 clips_per_video: 5 augmentation_config: train_crop_type: no_crop horizontal_flip_prob: 0.5 rgb_input_mean: [0.5] rgb_input_std: [0.5] val_center_crop: False
Training Parameters
- output_dir: Directory to save the results to
- encryption_key: Encryption key of the original model provided by nvidia
- model_config: configures the model settings
- model_type: specifies the type of model, rgb or opticalflow or both. Possible values: [rgb/of/joint]
- backbone: specifies the backbone network of model. Possible values:[resnet18/34/50/101/152]
- rgb_seq_length: specifies the length of RGB input sequence
- input_type: specifies the input type. Possible values:[2d/3d]
- sample_strategy: consecutive
- dropout_ratio: probability to drop the hidden units
- train_config: configure the training hyperparameters
- optim_config: configures parameters of optimiser like learning rate, scheduler, learning step etc.
- epochs: number of epochs to train for
- checkpoint_interval: interval between which we need to save the checkpoint
- dataset_config: configure the dataset
- train_dataset_dir: path to train data directory
- val_dataset_dir: path to test data directory
- label_map: map the class label to id
- output_shape
- batch_size: Specifies the batchsize.
- workers: number of workers that performs data loading, Usually the number equals the number of processors
- clips_per_video: number of clips to be sampled from single video
- augmentation_config: configure the augmentations to be used like crop, horizontal flip, normalisation etc.
Training / Fine tuning the model
# The paths are relative from inside the TAO Docker. No change required unless you changed `tao_mounts.json` file %env DATA_DIR = /data %env SPECS_DIR = /specs %env RESULTS_DIR = /results
!tao action_recognition train \ -e $SPECS_DIR/train_rgb_3d_finetune.yaml \ -r $RESULTS_DIR/rgb_3d_ptm \ -k $KEY \ model_config.rgb_pretrained_model_path=$RESULTS_DIR/pretrained/actionrecognitionnet_vtrainable_v1.0/resnet18_3d_rgb_hmdb5_32.tlt \ model_config.rgb_pretrained_num_classes=5
Rename the last checkpoint saved
!mv $HOST_RESULTS_DIR/rgb_3d_ptm/ar_model_epoch=19-val_loss=0.05.tlt $HOST_RESULTS_DIR/rgb_3d_ptm/rgb_only_model.tlt !ls -ltrh $HOST_RESULTS_DIR/rgb_3d_ptm/rgb_only_model.tlt
Evaluation, Inference and Exporting
We need to create a spec file in $HOST_SPECS_DIR folder for each of the three tasks Evaluation, Inference and Exporting. The files contain the following content
model_config: model_type: rgb backbone: resnet18 rgb_seq_length: 3 input_type: 3d sample_strategy: consecutive dropout_ratio: 0.0 dataset_config: label_map: fall_floor: 0 ride_bike: 1 output_shape: - 224 - 224 batch_size: 32 workers: 8 augmentation_config: train_crop_type: no_crop horizontal_flip_prob: 0.0 rgb_input_mean: [0.5] rgb_input_std: [0.5] val_center_crop: False
Save the file as evaluate_rgb.yaml, infer_rgb.yaml and export_rgb.yaml respectively.
Evaluating the finetuned model
Run the following command to start the evaluation.
!tao action_recognition evaluate \ -e $SPECS_DIR/evaluate_rgb.yaml \ -k $KEY \ model=$RESULTS_DIR/rgb_3d_ptm/rgb_only_model.tlt \ batch_size=1 \ test_dataset_dir=$DATA_DIR/test \ video_eval_mode=center
The output will be like this
100%|███████████████████████████████████████████| 60/60 [00:02<00:00, 27.19it/s] ******************************* fall_floor 96.67 ride_bike 100.0 ******************************* Total accuracy: 98.333 Average class accuracy: 98.333
Inference with the Finetuned model
For Inferencing run,
!tao action_recognition inference \ -e $SPECS_DIR/infer_rgb.yaml \ -k $KEY \ model=$RESULTS_DIR/rgb_3d_ptm/rgb_only_model.tlt \ inference_dataset_dir=$DATA_DIR/test/ride_bike \ video_inf_mode=center
The output will be like this
100%|███████████████████████████████████████████| 30/30 [00:01<00:00, 15.20it/s] /data/test/ride_bike/Yorki_Kassy_beim_Fahrrad_fahren_ride_bike_f_cm_np1_le_med_2 : ['ride_bike'] /data/test/ride_bike/Schuodde_kann_kein_Fahrrad_fahren_ride_bike_f_cm_np1_le_med_1 : ['ride_bike'] /data/test/ride_bike/Yorki_Kassy_beim_Fahrrad_fahren_ride_bike_f_cm_np1_le_med_0 : ['ride_bike'] /data/test/ride_bike/Fahrrad_fahren_mit_Albert_ride_bike_f_cm_np1_ba_med_0 : ['ride_bike'] /data/test/ride_bike/Fahrrad_fahren_mit_Albert_ride_bike_f_cm_np1_le_med_2 : ['ride_bike'] /data/test/ride_bike/Fahrrad_fahren_mit_Albert_ride_bike_f_cm_np1_ba_med_1 : ['ride_bike'] /data/test/ride_bike/Schuodde_kann_kein_Fahrrad_fahren_ride_bike_f_cm_np1_le_med_2 : ['ride_bike'] /data/test/ride_bike/lady_on_bike_ride_bike_f_cm_np1_ri_med_0 : ['ride_bike'] /data/test/ride_bike/Schuodde_kann_kein_Fahrrad_fahren_ride_bike_l_cm_np1_le_med_0 : ['ride_bike'] /data/test/ride_bike/Yorki_Kassy_beim_Fahrrad_fahren_ride_bike_f_cm_np1_fr_med_3 : ['ride_bike'] /data/test/ride_bike/Yorki_Kassy_beim_Fahrrad_fahren_ride_bike_f_cm_np1_ri_med_1 : ['ride_bike'] /data/test/ride_bike/lady_on_bike_ride_bike_f_cm_np1_ba_med_1 : ['ride_bike'] ...
Exporting the Model
Create an export directory
!mkdir -p $HOST_RESULTS_DIR/export
Run the following to start exporting
# Export the RGB model to encrypted ONNX model !tao action_recognition export \ -e $SPECS_DIR/export_rgb.yaml \ -k $KEY \ model=$RESULTS_DIR/rgb_3d_ptm/rgb_only_model.tlt\ output_file=$RESULTS_DIR/export/rgb_resnet18_3.etlt